October 2024: Generalizable Nonresponse Prediction and Machine Learning-based Adaptive Designs
Nonresponse is a critical issue for data quality in panel surveys. Many researchers have demonstrated the potential of machine learning (ML) models to predict nonresponse, enabling survey managers to intervene preemptively with low-propensity participants. We present a review of ML techniques for nonresponse propensity modeling in panel surveys, providing evidence that standard supervised learning models, using demographics and nonresponse history as predictors, consistently perform well in predicting nonresponse across various panel studies. Finally, we share early results from an experimental study to demonstrate how ML-based adaptive design might work in practice.
Christoph Kern is Junior Professor of Social Data Science and Statistical Learning at the Ludwig-Maximilians-University of Munich and Project Director at the Mannheim Centre for European Social Research (MZES). He received his PhD in social science (Dr. rer. pol.) from the University of Duisburg-Essen in 2016. Before joining LMU Munich, he was a Post-Doctoral Researcher at the Professorship for Statistics and Methodology at the University of Mannheim and Research Assistant Professor at the Joint Program in Survey Methodology (JPSM) at the University of Maryland. His work focuses on the reliable use of machine learning methods and new data sources in social science, survey research, and algorithmic fairness.
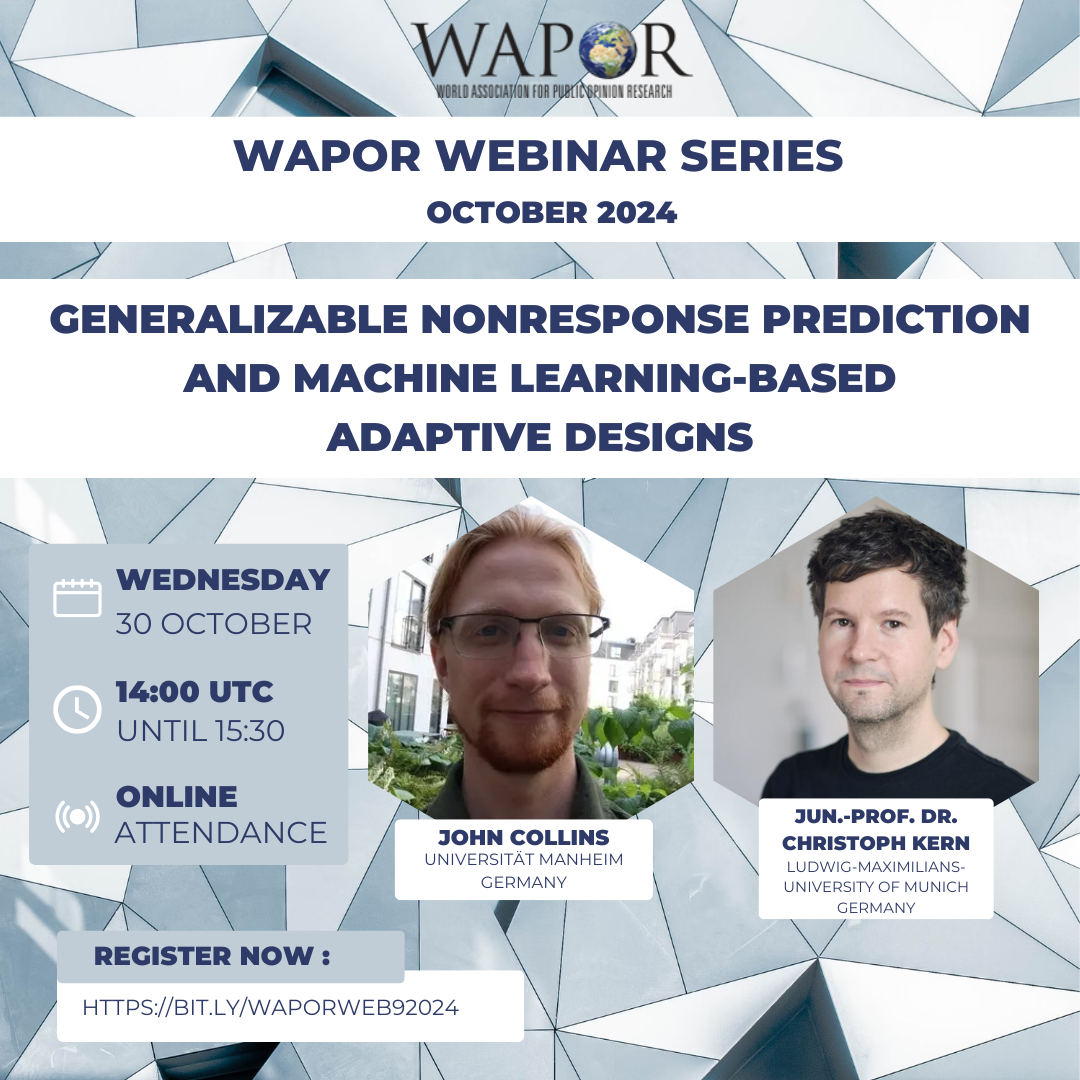